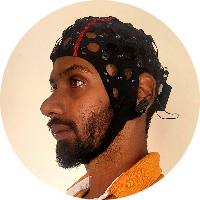
Rakesh Jakati
Project OwnerLeads project execution, ensuring seamless integration of EEG neurotechnology with AI. Manages team coordination, funding, and deployment. Oversees AI model enhancements and platform scalability.
Milestone Release 1 |
$8,000 USD | Pending | TBD |
Milestone Release 2 |
$7,000 USD | Pending | TBD |
Milestone Release 3 |
$17,000 USD | Pending | TBD |
Milestone Release 4 |
$8,000 USD | Pending | TBD |
Milestone Release 5 |
$10,000 USD | Pending | TBD |
Many individuals struggle with maintaining focus and interest at work, often feeling overwhelmed due to mental fatigue. Traditional self-assessments of focus levels are unreliable, leading people to believe they are productive when, in reality, their cognitive performance is declining. This results in frustration, decreased efficiency, and an overall drop in workplace well-being. Each individual varies in their optimal working patterns, and our AI agents help tailor focus strategies based on real-time brain activity. By leveraging wearable EEG technology, we ensure a highly personalized and adaptive approach to productivity enhancement.
New AI service
Our AI service uses EEG-based cognitive monitoring to track brain activity by analyzing focus levels and mental fatigue. By leveraging wearable EEG devices the AI detects engagement patterns classifies cognitive states and provides personalized recommendations. Using historical EEG data and reinforcement learning it refines insights to optimize productivity helping users work smarter and avoid burnout.
EEG data for analyzing brainwave patterns to assess focus and cognitive fatigue. It also integrates historical EEG data to track trends refine insights and provide more accurate assessments over time.
The AI generates personalized cognitive insights by analyzing EEG data. It informs users about their focus levels and productivity trends providing data-driven recommendations on when to work rest or adjust tasks for optimal efficiency.
The proposal for Productivity EEG-AI Agents is an ambitious workplace solution using AI for concentration optimization and reinforcement learning. It builds on an existing project funded in Deep Funding Round 4. While innovative and ethically conscious, it may face challenge with hardware dependence and privacy concerns regarding brain activity monitoring. Similar EEG headset solutions already exist in the market; however this is well researched and could transform workplace health.
New reviews and ratings are disabled for Awarded Projects
1. Recruit participants to collect new EEG data for refining the AI model. 2. Integrate advanced EEG feature extraction techniques to ensure better signal clarity. 3. Expand the existing dataset by collecting more sessions per user to improve model accuracy.
1. Anonymized EEG dataset collected from participants to enhance the existing AI model. 2. EEG data preprocessing pipeline with noise filtering feature extraction and anomaly detection. 3. A report on data acquisition methodology preprocessing techniques and initial observations.
$8,000 USD
1. Collect at least 100 high-quality EEG sessions. 2. Ensure dataset diversity across different cognitive conditions (engagement, fatigue, distraction).
1. Set up a structured EEG database to store and manage larger-scale data efficiently. 2. Data ingestion pipeline ensuring EEG recordings are seamlessly processed. 3. Refine feature extraction to identify more complex EEG signal variations.
1. EEG data pipeline for preprocessing storing and analyzing EEG signals. 2. Secure database setup to store EEG data while maintaining privacy. 3. Documentation of pipeline architecture data security measures and EEG signal processing techniques.
$7,000 USD
1. Ensure pipeline processes at least 100,000 EEG data points per user. 2. Scalable storage infrastructure supporting future EEG expansion. 3. Meet GDPR-compliant privacy standards for user data security.
1. Implement GRPPO-RL (Group Relative Policy Optimization Reinforcement Learning) to enhance model adaptability. 2. Train the RL model using the newly collected EEG data allowing it to dynamically adapt to individual user patterns. 3. Optimize the AI model by incorporating reinforcement learning ensuring improved cognitive state prediction.
1. GRPPO-RL enhanced EEG model improving EEG-based cognitive state prediction. 2. AI model that dynamically adapts to EEG variations for personalized recommendations. 3. Benchmark report comparing GRPPO-RL with traditional ML models.
$17,000 USD
1. AI model must adapt to individual EEG patterns with a self-learning accuracy of >70%. 2. Demonstrate real-time learning efficiency, improving response time in making recommendations.
1. Develop a user-friendly dashboard to visualize EEG insights and AI recommendations. 2. Conduct beta testing with users to gather feedback on AI-generated focus trends. 3. Refine the UX/UI to ensure ease of use and accessibility for a general audience.
1. Interactive dashboard displaying EEG-based cognitive insights trends and AI recommendations. 2. Beta testing program with real users for feedback and AI model validation. 3. Usability report summarizing user engagement feedback and dashboard performance improvements.
$8,000 USD
1. At least 80% of beta users must report satisfaction with the AI-generated insights. 2. Dashboard must visualize EEG features & cognitive trend analysis in an easy-to-understand format. 3. Ensure seamless EEG device integration with minimal user intervention.
1. Optimize the final version of the model based on real-world user feedback. 2. Deploy EEG-based AI system as service on SNET AI Marketplace.
1. AI service deployed and working on SNET platform. 2. Final project report summarizing system efficiency user adoption and future scalability plans.
$10,000 USD
1. Secure at least 50 real-world users to validate long-term effectiveness. 2. System must process and interpret EEG data with >80% accuracy in predicting cognitive states.
Please create account or login to post comments.
Reviews & Ratings
The proposal for Productivity EEG-AI Agents is an ambitious workplace solution using AI for concentration optimization and reinforcement learning. It builds on an existing project funded in Deep Funding Round 4. While innovative and ethically conscious, it may face challenge with hardware dependence and privacy concerns regarding brain activity monitoring. Similar EEG headset solutions already exist in the market; however this is well researched and could transform workplace health.
New reviews and ratings are disabled for Awarded Projects
© 2025 Deep Funding
Rakesh Jakati
Project Owner Mar 28, 2025 | 12:20 PMEdit Comment
Processing...
Please wait a moment.
Thank you for the thoughtful reviews. We appreciate the opportunity to clarify our approach to the concerns raised: 1. Hardware Agnosticism & Open Compatibility While we currently integrate with popular EEG devices like Muse and BrainBit for user accessibility, our architecture is device-agnostic. Raw data focus: We prioritize ADC accuracy over proprietary hardware ecosystems, allowing compatibility with any EEG device that provides raw waveform outputs. Open-source support: We actively validate our pipeline with open-source hardware OpenBCI) to ensure accessibility for cost-sensitive users and researchers. 2. Privacy & Ethical Data Control We acknowledge that real-time brain activity monitoring raises valid privacy concerns. Our solution is designed to: Zero data retention: Users retain full ownership of raw EEG data. We do not store or monetize brainwave patterns without explicit, revocable consent. Decentralized opt-in: Consent-based sharing (for research or wellness studies) uses blockchain-based permissions, ensuring transparency and user control. Federated Learning to minimize cloud dependence. This contrasts with centralized competitors who often sell user data to third parties. 3. Differentiation from Existing Solutions While commercial EEG apps exist, our project diverges in three key ways: Decentralized ownership: Competitors lock users into proprietary ecosystems; we empower users to own/export their data to third-party tools. Reinforcement learning (RL) personalization: Unlike static “scores,” our RL agents adapt to individual neurophysiological baselines, avoiding one-size-fits-all thresholds. Ethical monetization: Users can opt to contribute anonymized data (via tokenized incentives) to accelerate neurotech research, creating a community-driven alternative to corporate data exploitation.