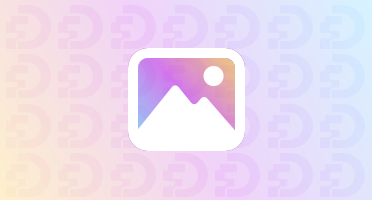
Knowledge Graph Construction from Raw Data
-
Type SingularityNET RFP
-
Funding Request n/a
-
RFP Guidelines Advanced knowledge graph tooling for AGI systems
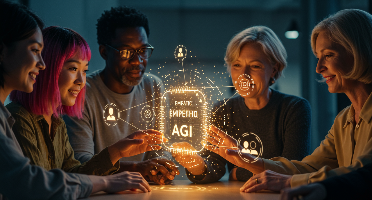
Coherence KG
-
Type SingularityNET RFP
-
Funding Request n/a
-
RFP Guidelines Advanced knowledge graph tooling for AGI systems
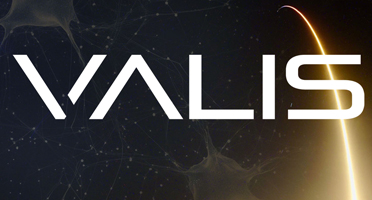
Quantum-Enhanced AGI: A Multi-Platform Evaluation
-
Type SingularityNET RFP
-
Funding Request n/a
-
RFP Guidelines Explore theoretical quantum computing models
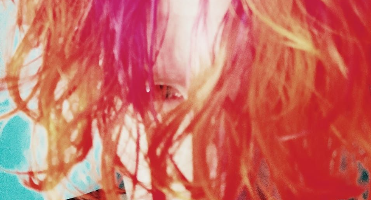
Post-Anthropocentric Framework for Adaptive AGI
-
Type SingularityNET RFP
-
Funding Request n/a
-
RFP Guidelines Develop a framework for AGI motivation systems

Loading results…